
Company Note: Google
Company Overview
Google (Alphabet Inc.) CEO: Sundar Pichai Corporate Address: 1600 Amphitheatre Parkway, Mountain View, CA 94043, USA Ticker Symbol: GOOGL Market: NASDAQ
Revenue History (in millions):
Year Revenue
2022 $282,836
2021 $257,637
2020 $182,527
Google is an American multinational technology company that specializes in Internet-related services and products, including online advertising, search engines, cloud computing, software, and hardware. As a subsidiary of Alphabet Inc., Google is one of the largest and most influential companies in the world, with a significant presence in various market segments.
Google's AI Offerings and Competitive Landscape
Google is a leading provider of AI solutions, with a comprehensive portfolio of products and services that span across the entire AI stack. The company's key strengths lie in its specialized AI hardware (Tensor Processing Units, or TPUs), widely adopted TensorFlow framework, comprehensive AI platform offerings, and extensive ecosystem for AI distribution and deployment.
Google's AI offerings compete with a range of companies, including established technology giants like IBM, NVIDIA, and Apple, as well as more specialized AI hardware providers like AMD and Graphcore. The following table summarizes Google's scores compared to these vendors:
Vendor Google Score Vendor Score Difference
IBM 4.5 3.2 1.3
AMD 4.5 2.7 1.8
NVIDIA 4.5 4.2 0.3
Apple 4.5 3.2 1.3
Graphcore 4.5 2.8 1.7
Google consistently scores higher than the other vendors, highlighting its strong position as an integrated AI stack provider. NVIDIA comes closest to Google, indicating that it is a strong competitor in the AI space, while IBM, Apple, AMD, and Graphcore have more focused offerings in specific layers of the AI stack.
Future Outlook and Growth Potential
Google's strong performance in the AI market is driven by its significant investments in research and development, as well as its ability to leverage its vast ecosystem of products and services to drive adoption of its AI solutions. The company's leadership in cloud computing, search, and digital advertising also provides a strong foundation for future growth in the AI space.
As the demand for AI-powered solutions continues to grow across industries, Google is well-positioned to capitalize on this trend. The company's comprehensive AI stack, coupled with its strong brand recognition and global presence, gives it a significant competitive advantage in the market.
However, Google also faces challenges from other large technology companies and specialized AI providers. To maintain its leadership position, the company will need to continue investing in research and development, expanding its AI ecosystem, and fostering partnerships with enterprises and developers.
Conclusion
Google is a dominant force in the AI industry, with a comprehensive portfolio of products and services that span across the entire AI stack. The company's strong scores compared to other vendors in the space highlight its leadership position and the breadth of its offerings.
As the AI market continues to evolve and grow, Google's strong foundation in cloud computing, search, and digital advertising, combined with its extensive AI capabilities, positions the company well for future success. However, to maintain its competitive edge, Google will need to continue innovating and adapting to the changing landscape of the AI industry.
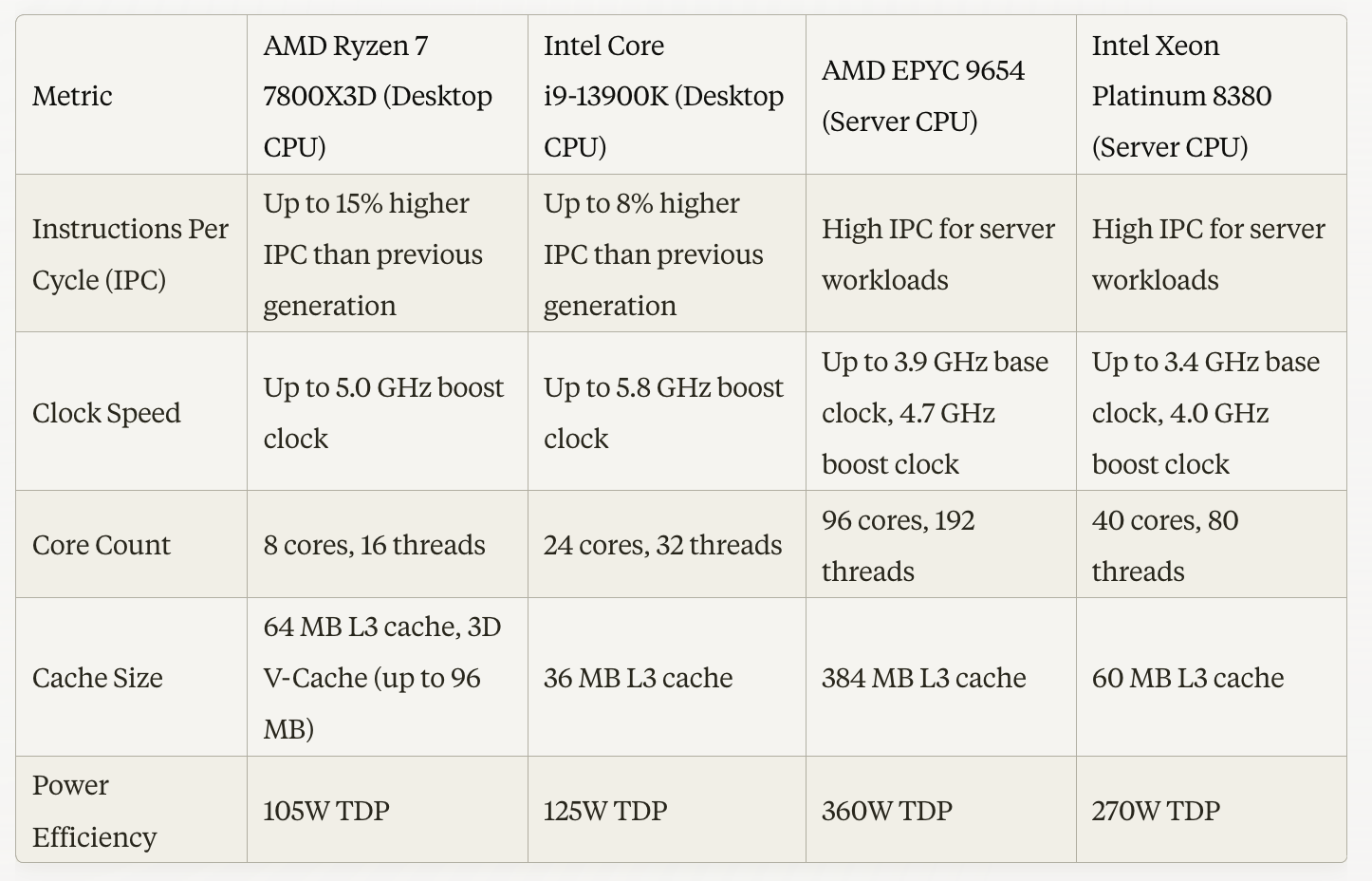
Company Note: Intel Corporation
Intel Corporation is an American multinational corporation and technology company headquartered in Santa Clara, California. Founded in 1968, Intel is one of the world's largest and most prominent semiconductor chip makers.
Products and Markets
Intel's primary products are microprocessors, chipsets, embedded processors, network interface controllers, flash memory, graphics chips, and many other devices related to computing and communications.
The company's processor products target several market segments:
Data Center: Intel's Xeon processors power most of the servers running cloud computing and enterprise data center infrastructure worldwide.
Client Computing: Intel's Core processors are found in most desktop and laptop computers across consumer and commercial markets.
Internet of Things (IoT): Intel's Atom and other low-power embedded processors enable smart and connected devices across industrial, retail, and consumer IoT applications.
Networking Solutions: Intel provides ethernet and telecommunications components for wired and wireless communications networks.
Programmable Solutions: Through its Altera acquisition, Intel offers field-programmable gate array (FPGA) products used in data centers, networking, automotive, and industrial applications.
Autonomous Driving: Intel's Mobileye business provides computer vision, machine learning, and data analytics solutions for advanced driver-assistance systems (ADAS) and autonomous vehicles.
Performance Metrics
Intel's processors and solutions are designed to deliver high performance, efficiency, and advanced capabilities across various computing workloads. Key performance metrics optimized by Intel include:
Instructions Per Cycle (IPC): Measuring the number of instructions a CPU can execute per clock cycle, indicating its processing efficiency.
Clock Speed: The frequency at which the processors operate, measured in gigahertz (GHz), with higher clock speeds generally indicating higher performance.
Core Count: The number of physical cores in the CPU, enabling parallel processing and improved multitasking performance.
Cache Size: The amount of high-speed memory cache available to the processor, which can significantly impact performance for specific workloads.
Power Efficiency: Evaluating the processors' performance-per-watt, which is crucial for various applications, including mobile devices and data centers.
Competitive Landscape
In the CPU market, Intel's primary competitor is Advanced Micro Devices (AMD). Other competitors include ARM-based processor makers like Qualcomm, Apple, Samsung, and various other fabless semiconductor companies in specific product segments.
Intel has long dominated the x86 CPU market for PCs and servers. However, it has faced increased competition from AMD's resurgence and the rise of ARM-based processors in data centers and other markets.
Market Position and Future Outlook
Intel is a market leader in many of its core product segments, including data center processors, desktop and laptop CPUs, and FPGA solutions. However, the company has faced challenges in recent years due to manufacturing delays, increased competition, and the shift towards specialized accelerators for emerging workloads like AI and machine learning.
Under CEO Pat Gelsinger, Intel is pursuing an "IDM 2.0" strategy, which includes significant investments in manufacturing capacity, advanced packaging technologies, and a renewed focus on product leadership and innovation.
The company aims to regain process leadership and expand into new growth opportunities, such as 5G networking, autonomous driving, and specialized accelerators for AI and high-performance computing (HPC).
Bottom Line
Intel Corporation is a semiconductor industry giant and a dominant force in the CPU and related chip markets. While facing intense competition and manufacturing challenges in recent years, the company remains a market leader in many segments.
Its ability to execute on its IDM 2.0 strategy, invest in advanced manufacturing capabilities, and deliver innovative products across its diverse portfolio will be crucial for maintaining its market position and capturing new growth opportunities in the rapidly evolving semiconductor industry.
Key Observations:
IPC (Instructions Per Cycle): Both AMD and Intel have made improvements in IPC with their latest generations, but AMD's Ryzen 7 7800X3D claims up to 15% higher IPC than the previous generation, outperforming Intel's Core i9-13900K.
Clock Speed: Intel's Core i9-13900K has a higher boost clock speed of up to 5.8 GHz compared to AMD's Ryzen 7 7800X3D at 5.0 GHz. However, for server CPUs, AMD's EPYC 9654 has a higher boost clock of 4.7 GHz compared to Intel's Xeon Platinum 8380 at 4.0 GHz.
Core Count: AMD's EPYC 9654 server CPU leads with 96 cores and 192 threads, while Intel's Xeon Platinum 8380 has 40 cores and 80 threads. For desktop CPUs, Intel's Core i9-13900K has a higher core count with 24 cores and 32 threads compared to AMD's Ryzen 7 7800X3D with 8 cores and 16 threads.
Cache Size: AMD's Ryzen 7 7800X3D has a larger combined cache size of up to 160 MB (64 MB L3 cache + 96 MB 3D V-Cache), while Intel's Core i9-13900K has 36 MB of L3 cache. For server CPUs, AMD's EPYC 9654 has a significantly larger 384 MB L3 cache compared to Intel's Xeon Platinum 8380 with 60 MB L3 cache.
Power Efficiency: AMD's desktop and server CPUs (Ryzen 7 7800X3D and EPYC 9654) have lower TDPs of 105W and 360W, respectively, compared to Intel's Core i9-13900K at 125W and Xeon Platinum 8380 at 270W, suggesting potentially better power efficiency for AMD's processors.

Company Note: AMD Advanced Micro Devices
Advanced Micro Devices (AMD) is an American multinational semiconductor company based in Santa Clara, California. Founded in 1969, AMD is one of the world's leading manufacturers of high-performance computing and graphics processors.
Products and Markets
AMD's primary products include:
Central Processing Units (CPUs):
Ryzen (desktop and laptop processors)
EPYC (server and data center processors)
Graphics Processing Units (GPUs):
Radeon (consumer and professional graphics)
Accelerators:
Instinct (AI and high-performance computing accelerators)
Performance Metrics
AMD's processors and accelerators are designed to deliver high performance and efficiency across various computing workloads. Key performance metrics optimized by AMD include:
TFLOPS (Tera Floating-Point Operations Per Second): Measuring the processors' floating-point performance for high-performance computing and AI workloads.
TOPS (Tera Operations Per Second): Measuring the overall computational throughput, including both floating-point and integer operations, for AI and machine learning tasks.
IPC (Instructions Per Cycle): Measuring the number of instructions a CPU can execute per clock cycle, indicating its processing efficiency.
Clock Speed: The frequency at which the processors operate, measured in gigahertz (GHz), with higher clock speeds generally indicating higher performance.
Power Efficiency: Evaluating the processors' performance-per-watt, which is crucial for various applications, including mobile devices and data centers.
AMD's products target several key markets, including client computing (desktop and laptop PCs), data center and cloud computing, gaming and professional visualization, artificial intelligence and machine learning, as well as high-performance computing (HPC).
Competitive Landscape
AMD's primary competitor in the CPU and GPU markets is Intel Corporation. Additionally, AMD competes with Nvidia in the GPU and accelerator space, as well as with various ARM-based processor manufacturers in specific markets like mobile and embedded devices.
AMD has made significant gains in recent years, challenging Intel's dominance in the CPU market with its high-performance Ryzen and EPYC processors based on the Zen architecture. The company has also strengthened its position in the GPU market with its Radeon and Instinct products.
Market Position and Future Outlook
AMD has successfully executed a remarkable turnaround under the leadership of CEO Dr. Lisa Su, regaining market share and establishing itself as a formidable competitor to Intel and Nvidia.
The company's focus on high-performance computing, data center solutions, and accelerators for emerging workloads like AI and machine learning has positioned it well for future growth opportunities.
However, AMD will need to continue investing in research and development, as well as manufacturing capabilities, to maintain its competitive edge and capitalize on the growing demand for powerful computing solutions across various markets.
Bottom Line
Advanced Micro Devices (AMD) is a leading semiconductor company that has made a strong comeback in recent years, challenging the dominance of industry giants like Intel and Nvidia. With its high-performance CPU and GPU products, as well as its focus on emerging areas like AI and HPC accelerators, AMD is well-positioned to capture growth opportunities in the rapidly evolving computing and data center markets. However, continued innovation, execution, and strategic investments will be crucial for AMD to sustain its momentum and solidify its position in the highly competitive semiconductor industry.

Company Report: Zhaoxin Semiconductor
Zhaoxin Semiconductor Co., Ltd. is a Chinese semiconductor company based in Shanghai, focused on developing x86-compatible processors and system-on-chip (SoC) solutions.
Products and Markets
Zhaoxin's primary products are x86-compatible central processing units (CPUs) designed for the domestic Chinese market. The company's CPU product lines include:
ZX-C series: Entry-level CPUs for desktop and mobile PCs
ZX-D series: Mid-range CPUs for desktops and servers
ZX-E series: High-performance CPUs for servers and workstations
In addition to CPUs, Zhaoxin also offers SoC solutions that integrate CPU, GPU, and other components on a single chip for various applications.
Performance Metrics
While specific performance metrics for Zhaoxin's CPUs are not publicly available, as an x86-compatible processor manufacturer, the company likely focuses on optimizing metrics similar to those used by Intel and AMD, such as:
Instructions Per Cycle (IPC): Measuring the number of instructions a CPU can execute per clock cycle, indicating its processing efficiency.
Clock Speed: The frequency at which the CPU operates, measured in gigahertz (GHz), with higher clock speeds generally indicating higher performance.
Core Count: The number of physical cores in the CPU, enabling parallel processing and improved multitasking performance.
Cache Size: The amount of high-speed memory cache available to the CPU, which can significantly impact performance for specific workloads.
Power Efficiency: Evaluating the CPU's performance-per-watt, which is crucial for various applications, including mobile devices and data centers.
Zhaoxin's target markets include desktop and laptop computers, servers and data centers, and embedded systems and Internet of Things (IoT) devices, primarily within the domestic Chinese market.
Competitive Landscape
Zhaoxin operates primarily in the Chinese domestic market, where it competes with international CPU manufacturers like Intel and AMD, as well as other domestic Chinese chip companies such as Loongson and Hygon.
Zhaoxin's focus on developing x86-compatible processors aims to reduce China's reliance on foreign technology and promote domestic semiconductor development. However, the company faces significant challenges in matching the performance and manufacturing capabilities of established players like Intel and AMD.
Market Position and Future Outlook
As a relatively new player in the CPU market, Zhaoxin has a limited market share and presence outside of China. Within the domestic Chinese market, the company has gained some traction by offering cost-effective x86-compatible solutions for various applications.
However, Zhaoxin will need to continue investing in research and development, as well as establishing partnerships and collaborations, to improve the performance and competitiveness of its products against international and domestic rivals.
The company's future growth prospects will depend on its ability to capitalize on the increasing demand for semiconductors in China, as well as the success of government initiatives to promote domestic chip production and reduce reliance on foreign technology.
Bottom Line
Zhaoxin Semiconductor is a Chinese company focused on developing x86-compatible CPUs and SoC solutions primarily for the domestic market. While still a relatively small player in the global semiconductor industry, Zhaoxin aims to contribute to China's efforts to develop a strong domestic chip manufacturing capability. However, the company faces significant challenges in terms of technology and manufacturing capabilities compared to established international competitors. Zhaoxin's success will depend on its ability to innovate, forge strategic partnerships, and leverage government support to gain a stronger foothold in the rapidly evolving semiconductor landscape.
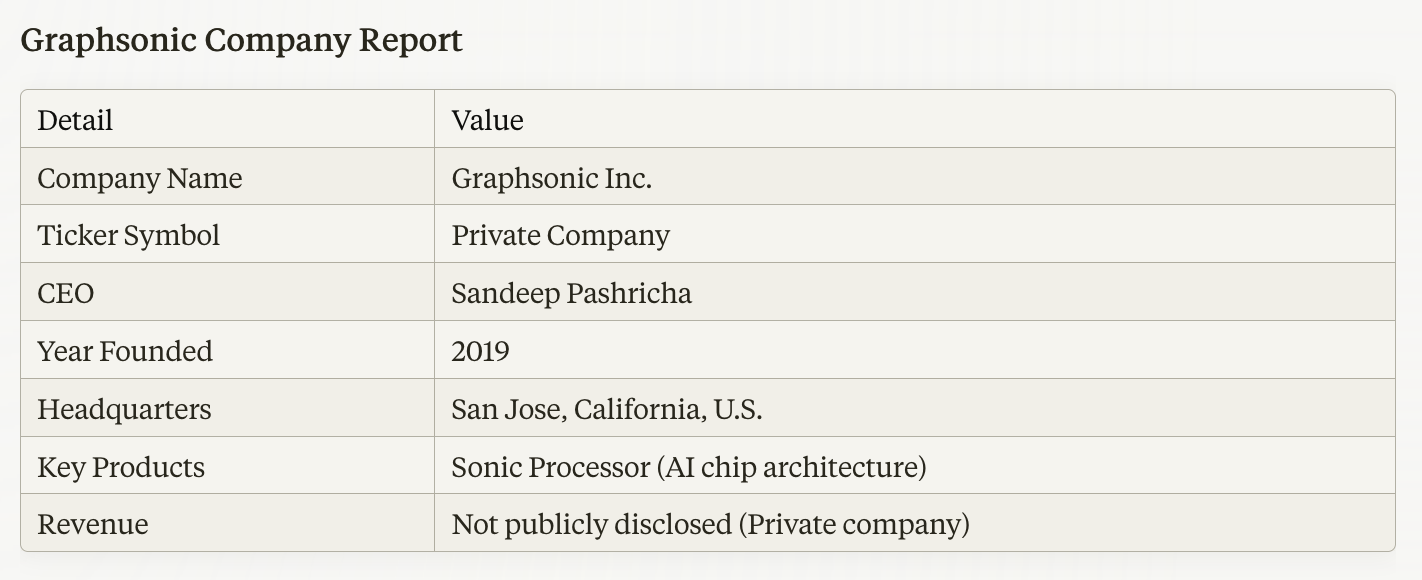
Company Note: Graphsonic
Graphsonic is a semiconductor startup based in San Jose, California, focused on developing a novel chip architecture called the Sonic Processor, designed for efficient artificial intelligence (AI) processing.
Products and Markets
Graphsonic's primary product is the Sonic Processor, a custom chip architecture optimized for AI and machine learning workloads. The Sonic Processor is designed to deliver high performance and energy efficiency for tasks such as neural network inference, training, and data processing.
Performance Metrics
While specific performance metrics for the Sonic Processor are not publicly available, as a novel AI chip architecture, Graphsonic likely aims to optimize metrics similar to other AI accelerators, such as:
TFLOPS (Tera Floating-Point Operations Per Second): Measuring the Sonic Processor's floating-point performance for AI and high-performance computing workloads.
TOPS (Tera Operations Per Second): Measuring the overall computational throughput, including both floating-point and integer operations, for AI and machine learning tasks.
Watts per TFLOP/TOPS (Energy Efficiency): Evaluating the energy efficiency of the Sonic Processor, which is crucial for data center and cloud environments.
Latency (Time to process a single input): Assessing the processor's low-latency capabilities for real-time AI applications like natural language processing and autonomous vehicles.
Throughput (Number of inputs processed per second): Measuring the processor's ability to handle high-volume AI workloads, such as training large language models or processing video streams.
Graphsonic aims to differentiate the Sonic Processor by delivering superior performance and energy efficiency for AI and machine learning workloads through its novel chip architecture.
The company's target markets include artificial intelligence (AI) and machine learning (ML), high-performance computing (HPC), data centers and cloud computing, autonomous vehicles and advanced driver assistance systems (ADAS), as well as Internet of Things (IoT) and edge computing.
Competitive Landscape
Graphsonic operates in the highly competitive AI chip market, where it faces competition from established players like Nvidia, Intel, AMD, and Qualcomm, as well as other startup companies developing custom AI accelerators, such as Cerebras Systems, Groq, and SambaNova Systems.
As a relatively new entrant, Graphsonic's differentiation lies in its novel Sonic Processor architecture, which the company claims can deliver superior performance and energy efficiency compared to existing AI chip designs.
Market Position and Future Outlook
As a startup, Graphsonic has yet to establish a significant market position in the AI chip industry. The company is currently focused on developing and refining its Sonic Processor technology, with plans to target various AI and high-performance computing applications.
Graphsonic's success will depend on its ability to demonstrate the advantages of its Sonic Processor architecture in terms of performance, efficiency, and scalability, as well as securing partnerships and customers in the highly competitive AI chip market.
Additionally, the company will need to navigate challenges such as securing adequate funding, attracting top talent, and establishing manufacturing and supply chain capabilities.
Bottom Line
Graphsonic is a startup company developing a novel AI chip architecture called the Sonic Processor, designed to deliver high performance and energy efficiency for AI and machine learning workloads. As a newcomer in the highly competitive AI chip market, Graphsonic faces significant challenges in establishing its technology and gaining market traction. The company's success will depend on its ability to execute on its innovative chip design, secure partnerships and customers, and navigate the complex landscape of the rapidly evolving AI chip industry.

Company Note: Ampere Computing
Ampere Computing is a semiconductor company based in Santa Clara, California, focused on developing Arm-based server processors and accelerators for cloud and edge computing applications. The company has received venture capital funding from prominent investors such as The Carlyle Group, Oracle, and NVIDIA.
Products and Markets
Ampere Computing's primary products are the Ampere Altra family of Arm-based server processors designed for cloud and edge computing workloads, offering high performance and energy efficiency. Additionally, the company offers the Ampere Altra Max series of AI/machine learning (ML) processors optimized for training and inference workloads in data centers and edge environments.
Performance Metrics
Ampere Computing's AI processors, such as the Ampere Altra Max series, are designed to deliver high performance and energy efficiency for AI and machine learning workloads. While specific performance metrics are not publicly available, the company likely focuses on optimizing metrics such as:
TFLOPS (Tera Floating-Point Operations Per Second): Measuring the processors' floating-point performance for AI and high-performance computing workloads.
TOPS (Tera Operations Per Second): Measuring the overall computational throughput, including both floating-point and integer operations, for AI and machine learning tasks.
Watts per TFLOP/TOPS (Energy Efficiency): Evaluating the energy efficiency of the processors, which is crucial for data center and cloud environments.
Latency (Time to process a single input): Assessing the processors' low-latency capabilities for real-time AI applications like natural language processing and autonomous vehicles.
Throughput (Number of inputs processed per second): Measuring the processors' ability to handle high-volume AI workloads, such as training large language models or processing video streams.
Ampere Computing likely aims to optimize these performance metrics to deliver competitive AI and machine learning performance while maintaining energy efficiency for cloud and edge computing environments.
Ampere's target markets include cloud service providers and hyperscalers, enterprise data centers, edge computing and 5G infrastructure, artificial intelligence and machine learning, as well as high-performance computing (HPC).
Competitive Landscape
In the Arm-based server processor market, Ampere Computing competes with established players like Marvell (through its acquisition of Cavium), Amazon (with its Graviton processors), and Huawei (with its Kunpeng processors). Additionally, Ampere faces competition from traditional x86 server CPU vendors like Intel and AMD, as well as other Arm-based processor companies targeting data center and edge computing applications.
In the AI/ML accelerator space, Ampere competes with companies like Nvidia, Intel, and various startups developing specialized AI chips.
Market Position and Future Outlook
As a relatively new entrant in the server and data center processor market, Ampere Computing has gained traction by offering high-performance, energy-efficient Arm-based solutions tailored for cloud and edge computing workloads. The company has secured partnerships and customers among major cloud service providers and hyperscalers, as well as in the enterprise and edge computing segments.
With its focus on Arm-based processors and AI/ML accelerators, Ampere is well-positioned to capitalize on the growing demand for energy-efficient and specialized computing solutions in data centers and edge environments.
However, the company will need to continue innovating and expanding its product portfolio to maintain its competitive edge against established players and emerging rivals in the rapidly evolving server and AI processor markets.
Bottom Line
Ampere Computing is a prominent player in the Arm-based server processor and AI accelerator markets, offering high-performance and energy-efficient solutions tailored for cloud, edge, and data center applications. With backing from major venture capital investors and its focus on emerging computing trends, Ampere has gained a foothold in the competitive server and AI processor landscapes. However, continued innovation, execution, and expanding its product offerings will be crucial for Ampere to solidify its market position and capture growth opportunities in these rapidly evolving segments.

Case Study: AI accelerators NVIDIA
AI accelerators NVIDIA Case Study
NVIDIA AI Processors: Performance Metrics
NVIDIA is a leading manufacturer of graphics processing units (GPUs) and specialized AI accelerators. Their flagship AI processors are the Tensor Core GPUs and the recently announced H100 GPU, designed for high-performance computing (HPC) and AI workloads.
1. TFLOPS (Tera Floating-Point Operations Per Second)
NVIDIA's AI processors deliver exceptional floating-point performance measured in TFLOPS. The A100 GPU, for instance, offers up to 312 TFLOPS of FP16 tensor core performance, while the more recent H100 GPU boasts up to 500 TFLOPS of FP16 tensor core performance.
2. TOPS (Tera Operations Per Second)
In addition to TFLOPS, NVIDIA also reports TOPS figures for their AI processors, which measure overall computational throughput, including both floating-point and integer operations. The A100 GPU delivers up to 19.5 TOPS of INT8 performance, while the H100 GPU offers up to 60 TOPS of INT8 performance.
3. Watts per TFLOP/TOPS (Energy Efficiency)
Energy efficiency is a crucial factor for AI accelerators, especially in data centers and cloud environments. NVIDIA has made significant strides in improving the performance-per-watt of their AI processors. The A100 GPU delivers up to 20 TFLOPS per watt (FP16) and up to 1.25 TOPS per watt (INT8). The H100 GPU further improves on this, offering up to 30 TFLOPS per watt (FP16) and up to 1.8 TOPS per watt (INT8).
4. Latency (Time to process a single input)
Low latency is essential for real-time AI applications, such as natural language processing, recommendation systems, and autonomous vehicles. NVIDIA's AI processors are designed to provide low-latency inference capabilities. For example, the A100 GPU can process a single input in as little as 2.8 milliseconds for computer vision workloads, while the H100 GPU promises even lower latency, although specific figures are not yet available.
5. Throughput (Number of inputs processed per second)
Throughput is a critical metric for batch processing and high-volume AI workloads, such as training large language models or processing video streams. NVIDIA's AI processors excel in this area, with the A100 GPU capable of processing up to 1.6 billion images per second for computer vision tasks. The H100 GPU is expected to deliver even higher throughput, with NVIDIA claiming up to 3.5 times higher performance compared to the A100 for certain AI workloads.
In summary, NVIDIA's AI processors, including the A100 and H100 GPUs, offer exceptional performance across TFLOPS, TOPS, energy efficiency, latency, and throughput metrics. These capabilities make NVIDIA's AI accelerators well-suited for a wide range of AI applications, from high-performance training to low-latency inference tasks.
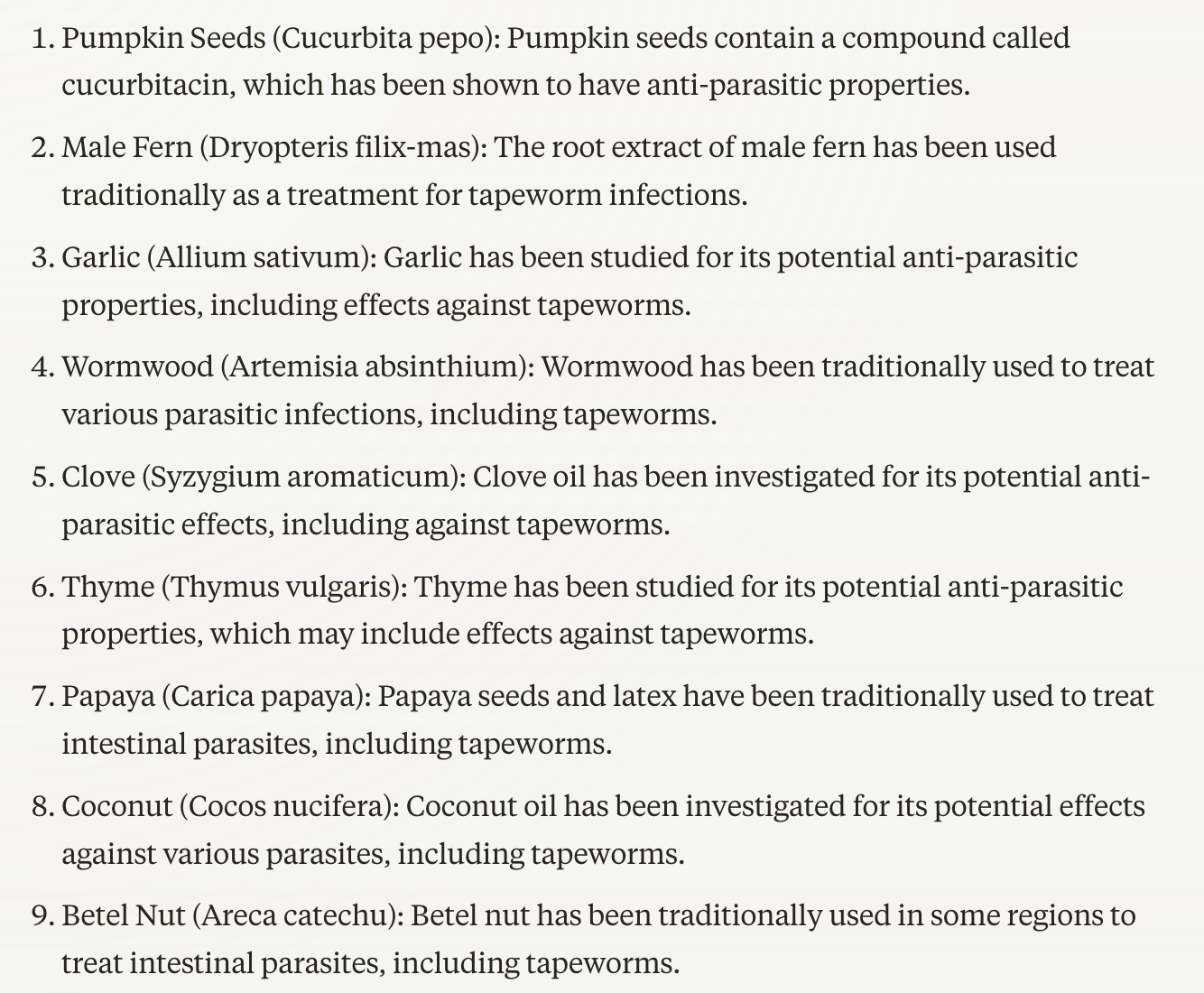
Key Issue: What food assists with getting rid of tape worms ?
There are several medicinal plants that have been traditionally used or studied for their potential effects against tapeworms. However, it's important to note that self-treatment of tapeworm infections is not recommended, and proper medical advice should be sought. Here are some of the known medicinal plants:
Pumpkin Seeds (Cucurbita pepo): Pumpkin seeds contain a compound called cucurbitacin, which has been shown to have anti-parasitic properties.
Male Fern (Dryopteris filix-mas): The root extract of male fern has been used traditionally as a treatment for tapeworm infections.
Garlic (Allium sativum): Garlic has been studied for its potential anti-parasitic properties, including effects against tapeworms.
Wormwood (Artemisia absinthium): Wormwood has been traditionally used to treat various parasitic infections, including tapeworms.
Clove (Syzygium aromaticum): Clove oil has been investigated for its potential anti-parasitic effects, including against tapeworms.
Thyme (Thymus vulgaris): Thyme has been studied for its potential anti-parasitic properties, which may include effects against tapeworms.
Papaya (Carica papaya): Papaya seeds and latex have been traditionally used to treat intestinal parasites, including tapeworms.
Coconut (Cocos nucifera): Coconut oil has been investigated for its potential effects against various parasites, including tapeworms.
Betel Nut (Areca catechu): Betel nut has been traditionally used in some regions to treat intestinal parasites, including tapeworms.

AI accelerators
AI accelerators play a crucial role in the artificial intelligence market by providing specialized hardware designed to speed up AI workloads, particularly in the areas of machine learning, deep learning, and neural network processing. These accelerators offer significant performance improvements and energy efficiency compared to general-purpose CPUs, enabling faster training and inference of AI models.
Market Size and Growth:
According to a report by MarketsandMarkets, the global AI accelerator market size is expected to grow from USD 11.6 billion in 2020 to USD 72.6 billion by 2025, at a Compound Annual Growth Rate (CAGR) of 44.2% during the forecast period. This growth is driven by the increasing demand for AI-powered applications across various industries, the need for high-performance computing, and the growing volume of data generated by IoT devices and other sources.
Companies and Industries:
Several companies, including technology giants and specialized AI chip manufacturers, have developed AI accelerators. Some of the key players in the market include:
-table above-
Tangible Benefits and Metrics:
AI accelerators offer several tangible benefits across different industries:
Faster Training and Inference: AI accelerators significantly reduce the time required to train and run AI models, enabling quicker development and deployment of AI applications.
Improved Performance: Specialized hardware delivers superior performance compared to general-purpose CPUs, allowing for more complex and accurate AI models.
Energy Efficiency: AI accelerators are designed to be more energy-efficient than CPUs, reducing power consumption and associated costs.
Scalability: AI accelerators enable organizations to scale their AI workloads efficiently, accommodating growing data volumes and complexity.
Real-time Processing: In industries such as automotive and healthcare, AI accelerators enable real-time processing of data, critical for applications like autonomous vehicles and medical diagnosis.
Metrics used to evaluate the performance of AI accelerators include:
TFLOPS (Tera Floating-Point Operations Per Second)
TOPS (Tera Operations Per Second)
Watts per TFLOP or TOPS (Energy Efficiency)
Latency (Time to process a single input)
Throughput (Number of inputs processed per second)
Consolidators and Adoption:
Large technology companies like NVIDIA, Intel, and Google are consolidating the AI accelerator market through acquisitions and in-house development. These companies leverage their AI accelerators to offer AI-powered services and platforms, driving adoption across various industries.
Industries adopting AI accelerators include:
Cloud Computing and Data Centers
Automotive and Autonomous Vehicles
Healthcare and Medical Imaging
Finance and Fraud Detection
Retail and Personalized Recommendations
Manufacturing and Predictive Maintenance
Telecommunications and 5G Networks
Aerospace and Defense
Gaming and Entertainment
Smart Cities and Infrastructure
As AI continues to permeate various sectors, the demand for AI accelerators is expected to grow, driving further innovation and market consolidation.
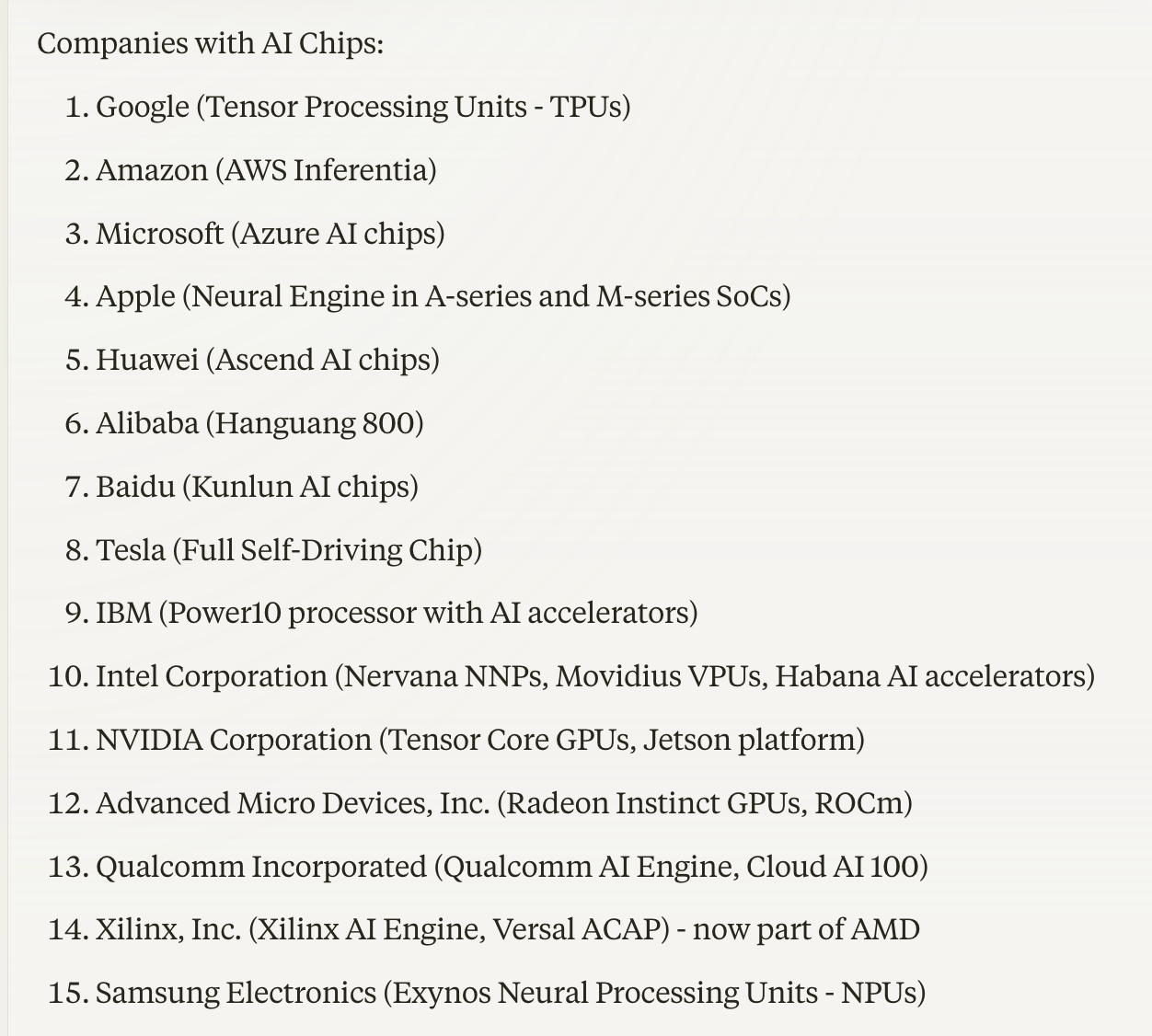
A.I. Chips
Recommended soundtrack: Bright lights big city, Gary Clark, Jr.
The AI Chip Industry: Future Direction and Market Adoption
Introduction
The artificial intelligence (AI) chip industry has experienced rapid growth in recent years, driven by the increasing demand for AI-powered applications across various sectors. This report examines the current state of the AI chip market, analyzes product announcements from key players, and explores the future direction of the industry based on the adoption of different layers within the AI ecosystem.
Market Overview
The AI chip market comprises a diverse range of companies, including technology giants like Google, Amazon, Microsoft, Apple, and NVIDIA, as well as specialized AI chip manufacturers such as Graphcore, Cerebras Systems, and Habana Labs (now part of Intel). These companies offer a variety of AI accelerators, such as GPUs, ASICs, FPGAs, and custom-designed chips, catering to different segments of the AI market.
Product Announcements and Ecosystem Adoption
Recent product announcements from major players in the AI chip industry reveal a focus on developing hardware solutions for the lower layers of the AI ecosystem, particularly AI chips and hardware infrastructure. Companies like NVIDIA, Intel, AMD, and Google have introduced powerful AI accelerators and platforms designed to handle the compute-intensive tasks of AI workloads, such as deep learning and machine learning.
The emphasis on AI chips and hardware infrastructure suggests that this layer of the AI ecosystem is being filled first, creating a market wave of adoption and spurring competition among chip manufacturers. As more companies develop and optimize their AI hardware offerings, it is likely that this trend will continue in the near future, driving innovation and performance improvements in AI accelerators.
Gaps in the AI Ecosystem and Future Opportunities
While the AI chip and hardware infrastructure layer has seen significant development and adoption, other layers of the AI ecosystem, such as AI frameworks and libraries, AI algorithms and models, and AI application and integration, have not yet received the same level of attention from chip manufacturers.
This presents an opportunity for companies to differentiate themselves by developing solutions that address the higher layers of the AI stack. For example, creating optimized AI frameworks and libraries that can take full advantage of the capabilities of AI accelerators could help bridge the gap between hardware and software, making it easier for developers to build and deploy AI applications.
Similarly, investing in the development of AI algorithms and models that are specifically designed to run efficiently on AI chips could provide a competitive edge for companies looking to offer end-to-end AI solutions. By focusing on the higher layers of the AI ecosystem, chip manufacturers can create a more comprehensive and integrated AI stack, facilitating the adoption of AI technologies across industries.
Companies Best Positioned for Future Development
Among the key players in the AI chip industry, companies with strong expertise in both hardware and software development are well-positioned to address the gaps in the AI ecosystem and drive future growth. NVIDIA, Intel, and Google, for example, have extensive experience in developing AI accelerators, as well as creating software frameworks and tools for AI development.
These companies have the resources and knowledge to create integrated AI solutions that span multiple layers of the AI stack, from hardware infrastructure to frameworks, algorithms, and applications. By leveraging their expertise and ecosystem partnerships, they can help bridge the gap between the lower and higher layers of the AI ecosystem, enabling faster and more widespread adoption of AI technologies.
Conclusion
The AI chip industry is poised for continued growth and innovation, driven by the increasing demand for AI-powered applications across various sectors. While the current focus on AI chips and hardware infrastructure has created a market wave of adoption, there are still opportunities for companies to differentiate themselves by addressing the higher layers of the AI ecosystem.
As the industry evolves, companies that can develop integrated AI solutions spanning multiple layers of the AI stack will be well-positioned to lead the market and drive the future direction of AI adoption. By investing in the development of optimized AI frameworks, algorithms, and applications, these companies can help bridge the gap between hardware and software, facilitating the widespread deployment of AI technologies across industries.
Google (Tensor Processing Units - TPUs): Google's TPUs are custom-built AI accelerators designed for deep learning and machine learning workloads. They offer high performance and energy efficiency for training and inference tasks. Google uses TPUs in its data centers to power various AI-driven services, such as Google Search, Google Translate, and Google Photos. Google also offers TPUs through its Google Cloud Platform, enabling developers and businesses to accelerate their AI workloads in the cloud.
Amazon (AWS Inferentia): AWS Inferentia is a custom-built AI chip designed for high-performance machine learning inference. It offers low latency and high throughput, making it suitable for real-time AI applications. AWS Inferentia is integrated into Amazon's EC2 Inf1 instances, providing customers with a cost-effective and scalable solution for deploying AI models in the cloud. It is also supported by popular machine learning frameworks, such as TensorFlow and PyTorch, and can be used with AWS's SageMaker platform for end-to-end machine learning workflows.
Microsoft (Azure AI chips): Microsoft has developed custom AI chips for its Azure cloud platform, including the Rapid Prototyping Platform (RPP) and the Holographic Processing Unit (HPU) for HoloLens. These chips are designed to accelerate AI workloads and provide high performance for specific applications, such as computer vision and natural language processing. Microsoft also collaborates with Intel, NVIDIA, and Graphcore to offer a range of AI accelerators on Azure, catering to diverse customer needs.
Apple (Neural Engine in A-series and M-series SoCs): Apple's Neural Engine is a dedicated AI accelerator integrated into its A-series and M-series systems-on-chip (SoCs). The Neural Engine is designed to accelerate machine learning tasks on Apple devices, such as the iPhone, iPad, and Mac. It enables fast and efficient execution of AI models for applications like face recognition, natural language processing, and augmented reality. Apple's Core ML framework allows developers to leverage the Neural Engine's capabilities to build AI-powered apps for iOS, iPadOS, and macOS.
Huawei (Ascend AI chips): Huawei's Ascend AI chips are designed for a wide range of AI applications, from edge devices to data centers. The Ascend series includes the Ascend 310, 910, and 610 chips, which offer high performance and energy efficiency for AI inference and training tasks. Huawei's AI chips are integrated into its smartphones, tablets, and other consumer devices, as well as its enterprise offerings, such as servers and cloud services. The company also provides the MindSpore AI computing framework, which is optimized for Ascend chips and enables developers to build and deploy AI applications easily.
Alibaba (Hanguang 800): Alibaba's Hanguang 800 is a custom-built AI chip designed for high-performance machine learning inference in cloud and edge computing scenarios. It offers high efficiency and low latency for tasks such as image and video analysis, natural language processing, and recommendation systems. The Hanguang 800 is used in Alibaba's cloud computing infrastructure to power its AI services, such as Alibaba Cloud's ET Brain and Alibaba DAMO Academy's research projects.
Baidu (Kunlun AI chips): Baidu's Kunlun AI chips are designed for a variety of AI workloads, including deep learning, machine learning, and edge computing. The Kunlun series includes the Kunlun 1 and Kunlun 2 chips, which offer high performance and flexibility for different application scenarios. Baidu uses Kunlun chips in its data centers to power its AI-driven services, such as Baidu Search, Baidu Maps, and DuerOS virtual assistant. The company also offers Kunlun chips to external customers through its Baidu Cloud AI platform.
Tesla (Full Self-Driving Chip): Tesla's Full Self-Driving (FSD) Chip is a custom-designed AI accelerator specifically developed for autonomous driving tasks. It provides high performance and low latency for processing sensor data, such as camera feeds and radar signals, and making real-time decisions for vehicle control. The FSD Chip is integrated into Tesla's vehicles, enabling advanced driver assistance features and laying the foundation for fully autonomous driving capabilities in the future.
IBM (Power10 processor with AI accelerators): IBM's Power10 processor includes built-in AI accelerators, which are designed to speed up machine learning and deep learning workloads. The Power10 processor offers high performance and energy efficiency for AI applications in data centers and cloud environments. IBM also provides the PowerAI software toolkit, which includes optimized libraries and frameworks for AI development on Power systems. The Power10 processor and PowerAI toolkit are used by IBM's customers and partners to build and deploy AI solutions across various industries.
Intel Corporation (Nervana NNPs, Movidius VPUs, Habana AI accelerators): Intel offers a range of AI accelerators, including the Nervana Neural Network Processors (NNPs), Movidius Vision Processing Units (VPUs), and Habana AI accelerators. The Nervana NNPs are designed for high-performance deep learning training and inference in data centers, while the Movidius VPUs are optimized for low-power AI inference in edge devices. The Habana AI accelerators, acquired by Intel in 2019, offer high efficiency and scalability for both training and inference workloads. Intel's AI accelerators are supported by the oneAPI toolkit and OpenVINO toolkit, enabling developers to build and optimize AI applications across different hardware platforms.
NVIDIA Corporation (Tensor Core GPUs, Jetson platform): NVIDIA's Tensor Core GPUs are designed to accelerate AI workloads, particularly deep learning tasks. They offer high performance and energy efficiency for training and inference in data centers, cloud environments, and edge devices. NVIDIA's Jetson platform is a series of embedded systems-on-module (SoMs) that integrate Tensor Core GPUs, providing a compact and power-efficient solution for AI inference in edge devices and autonomous systems. NVIDIA also provides the CUDA parallel computing platform and cuDNN library, which enable developers to build and optimize AI applications for NVIDIA GPUs.
Advanced Micro Devices, Inc. (Radeon Instinct GPUs, ROCm): AMD's Radeon Instinct GPUs are designed for high-performance computing and AI workloads in data centers and cloud environments. They offer high throughput and energy efficiency for deep learning training and inference tasks. AMD also provides the ROCm (Radeon Open Compute) platform, an open-source software stack for GPU computing that includes optimized libraries and frameworks for AI development. ROCm enables developers to build and deploy AI applications on AMD GPUs using popular machine learning frameworks like TensorFlow and PyTorch.
Qualcomm Incorporated (Qualcomm AI Engine, Cloud AI 100): Qualcomm's AI Engine is a suite of hardware and software components designed to accelerate AI workloads on Qualcomm Snapdragon mobile platforms. It includes the Hexagon Vector Processor, Adreno GPU, and Kryo CPU, which work together to provide efficient AI processing for tasks like computer vision, natural language processing, and sensor fusion. Qualcomm also offers the Cloud AI 100 accelerator, which is designed for high-performance AI inference in data centers and edge servers. The Cloud AI 100 provides low latency and high throughput for real-time AI applications and services.
Xilinx, Inc. (Xilinx AI Engine, Versal ACAP) - now part of AMD: Xilinx's AI Engine is a flexible and adaptable hardware architecture designed for high-performance AI inference and signal processing. It is part of Xilinx's Versal Adaptive Compute Acceleration Platform (ACAP), which combines scalar processing engines, adaptable hardware engines, and intelligent engines to provide a highly efficient and customizable solution for AI workloads. The Xilinx AI Engine and Versal ACAP are used in a variety of applications, including automotive, aerospace and defense, and data center markets. Xilinx also provides the Vitis AI development platform, which enables developers to optimize and deploy AI models on Xilinx hardware.
Samsung Electronics (Exynos Neural Processing Units - NPUs): Samsung's Exynos Neural Processing Units (NPUs) are dedicated AI accelerators integrated into the company's Exynos mobile processors. They are designed to provide high performance and energy efficiency for AI workloads on Samsung's smartphones, tablets, and other mobile devices. The Exynos NPUs enable fast and efficient execution of AI models for applications like face recognition, object detection, and natural language processing. Samsung also provides the Exynos NN software development kit (SDK), which allows developers to leverage the Exynos NPU's capabilities to build AI-powered mobile apps.
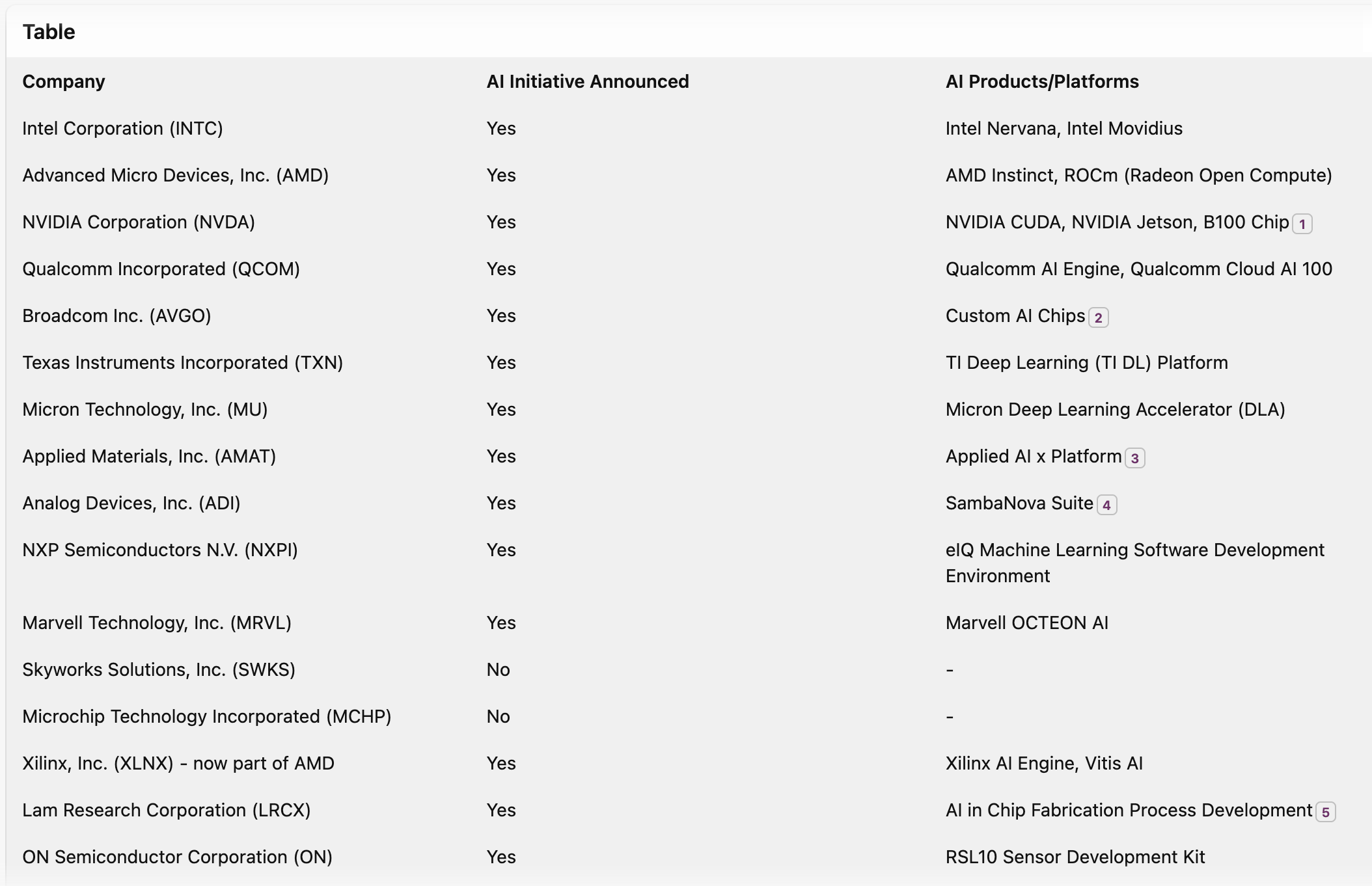
Key Issue: Can you help me understand the semiconductor industry ?
Recommended soundtrack: Who are you, The Who
The Semiconductor Industry: An Overview
The semiconductor industry is a complex and dynamic ecosystem that plays a crucial role in the development and advancement of modern electronics. This industry encompasses a wide range of segments, each contributing to the creation of cutting-edge technologies that power our digital world. From artificial intelligence (AI) processors and graphics processing units (GPUs) to memory solutions and radio frequency (RF) devices, the semiconductor industry offers a diverse array of products and services.
Market Segmentation and Growth
The semiconductor industry can be divided into various segments based on the types of products and their applications. Some of the key segments include:
AI Processors
GPUs and Accelerators
Mobile System-on-Chips (SoCs) and AI Accelerators
Networking and Broadband Solutions
Memory Solutions
Semiconductor Manufacturing Equipment
Field-Programmable Gate Arrays (FPGAs) and Adaptive SoCs
Image Sensors and Power Management Solutions
RF Solutions
Each of these segments has shown significant growth potential in recent years, driven by the increasing demand for faster, smarter, and more efficient electronic devices.
AI Processors and GPUs
AI processors, designed specifically for artificial intelligence applications, have emerged as one of the fastest-growing segments in the semiconductor industry. In 2020, the AI processor market was valued at USD 7.2 billion and is expected to grow at a compound annual growth rate (CAGR) of 35.0% from 2021 to 2028. This growth is primarily driven by the increasing demand for AI-powered devices and services across various industries, such as automotive, healthcare, and consumer electronics.
Similarly, GPUs and accelerators, which are essential for graphics-intensive tasks and high-performance computing, have shown significant growth potential. The global GPU market is projected to reach USD 200.9 billion by 2027, growing at a CAGR of 33.6% during the forecast period.
Mobile SoCs and AI Accelerators
The mobile SoC and AI accelerator market, which is crucial for enabling AI capabilities on edge devices, is another promising segment. This market is projected to reach a size of USD 15.8 billion by 2028, growing at a CAGR of 22.6% from 2021 to 2028. The growth of this segment is fueled by the increasing adoption of AI-enabled smartphones, tablets, and other mobile devices.
Memory Solutions and Semiconductor Manufacturing Equipment
Memory solutions, including DRAM and flash memory, are essential components in virtually all electronic devices. The global semiconductor memory market is projected to reach USD 162.3 billion by 2025, growing at a CAGR of 7.7% during the forecast period. The growth of this segment is driven by the increasing demand for high-performance and high-capacity memory solutions in data centers, smartphones, and other electronic devices.
The semiconductor manufacturing equipment market, which is vital for the production of advanced chips, is expected to reach USD 142.9 billion by 2028, growing at a CAGR of 9.8% from 2021 to 2028. The growth of this segment is driven by the increasing demand for advanced semiconductor fabrication processes and the need for high-performance computing solutions.
Other Segments
Other segments, such as networking and broadband solutions, FPGAs and adaptive SoCs, image sensors and power management solutions, and RF solutions, are also expected to witness significant growth in the coming years. The growth of these segments is driven by various factors, such as the increasing adoption of 5G networks, the proliferation of Internet of Things (IoT) devices, and the growing demand for energy-efficient electronic devices.
Corporate Introductions and Product Innovations
The semiconductor industry has witnessed a remarkable evolution over the past few decades, with corporate introductions and product innovations shaping its trajectory. Some of the key players in the industry include:
Company Founded Key Products/Technologies
Intel 1968 CPUs, SoCs, AI Processors
AMD 1969 CPUs, GPUs, SoCs
NVIDIA 1993 GPUs, AI Processors, Accelerators
Qualcomm 1985 Mobile SoCs, AI Accelerators, RF Solutions
Broadcom 1961 Networking Solutions, Broadband Chips
Texas Instruments 1930 Analog ICs, Embedded Processors, DSPs
These companies have been at the forefront of innovation in the semiconductor industry, constantly pushing the boundaries of performance, power efficiency, and integration.
Over the years, the semiconductor industry has witnessed a shift from discrete components and simple integrated circuits to more complex SoCs that integrate multiple functions on a single chip. The introduction of GPUs in the late 1990s by companies like NVIDIA and AMD revolutionized graphics processing and paved the way for accelerated computing. The early 2000s saw the rise of mobile SoCs, with companies like Qualcomm and Apple leading the way in integrating powerful processing capabilities into small, energy-efficient packages.
In recent years, the focus has shifted towards the development of specialized AI accelerators and processors, designed to handle the unique demands of machine learning and deep learning workloads. Companies like NVIDIA, Intel, and Google have introduced dedicated AI chips, such as the NVIDIA Tensor Core GPUs, Intel Nervana Neural Network Processors (NNPs), and Google Tensor Processing Units (TPUs).
Artificial Intelligence and Future Direction
The integration of AI capabilities into semiconductor products has been a major theme in recent years. Companies across the industry have made significant announcements regarding their AI initiatives and product offerings.
Company AI Initiative AI Products/Platforms
NVIDIA Yes CUDA, Tensor Core GPUs, Jetson Platform
Intel Yes Nervana NNPs, Movidius VPU, Habana AI Accelerators
AMD Yes Radeon Instinct GPUs, ROCm (Radeon Open Compute)
Qualcomm Yes Qualcomm AI Engine, Cloud AI 100
Xilinx (AMD) Yes Xilinx AI Engine, Versal ACAP
Google Yes Tensor Processing Units (TPUs)
Apple Yes Neural Engine in A-series and M-series SoCs
The future direction of the semiconductor industry is deeply intertwined with advancements in AI and the increasing demand for AI-enabled devices and services across various sectors. The development of specialized AI accelerators and processors, the integration of AI capabilities into edge devices, and the convergence of AI with other emerging technologies, such as 5G networks and IoT, will shape the future of the industry.
In addition to hardware innovations, the industry is also focusing on the development of software tools and frameworks that simplify AI development and deployment. Initiatives like NVIDIA's CUDA platform, Intel's oneAPI, and AMD's ROCm aim to provide developers with a unified programming model across diverse hardware architectures, facilitating the creation of AI applications that can run efficiently on various devices.
Conclusion
The semiconductor industry is a critical enabler of technological advancement in the digital age. As the demand for faster, smarter, and more efficient electronic devices continues to grow, the industry is poised for significant growth in the coming years. The industry's ability to deliver cutting-edge solutions across a wide range of applications, from consumer electronics to industrial automation, positions it as a key driver of innovation and economic growth.
The future of the semiconductor industry is closely tied to advancements in AI and the increasing demand for AI-enabled devices and services. The industry's focus on specialized AI hardware, edge computing, convergence with emerging technologies, and software tools and frameworks will define its trajectory in the coming years. As the industry continues to evolve and innovate, it will play a crucial role in shaping the future of technology and enabling the widespread adoption of AI across diverse applications.

Key Issue: Could you explain the market opportunity within the Geothermal power market ?
Recommended artist: Son House
—————-
Geothermal Market Opportunity by State:
California:
The Salton Sea KGRA, located near Calipatria, has an estimated resource of 2,500 MW and an annual revenue potential of $2.37 billion. The area could support 405 wells, with a current well cost of $2.03 billion (at $5M per well) or $4.05 billion (at $10M per well). This KGRA is known for its high-temperature resources and potential for lithium extraction.
The Coso KGRA, near Ridgecrest, has an estimated resource of 750 MW and an annual revenue potential of $709.56 million. It could support 122 wells, with a current well cost of $610 million (at $5M per well) or $1.22 billion (at $10M per well). Coso is notable for its high-temperature resources and existing power plants.
The Geysers KGRA, close to Healdsburg, has an estimated resource of 1,500 MW and an annual revenue potential of $1.42 billion. The area could support 243 wells, with a current well cost of $1.22 billion (at $5M per well) or $2.43 billion (at $10M per well). The Geysers is the world's largest geothermal field, with a long history of power production.
Nevada:
The Beowawe KGRA, near the town of Beowawe, has an estimated resource of 150 MW and an annual revenue potential of $141.91 million. It could support 24 wells, with a current well cost of $120 million (at $5M per well) or $240 million (at $10M per well). Beowawe is known for its high-temperature resources and existing power plant.
Utah:
The Roosevelt Hot Springs KGRA, near Milford, has an estimated resource of 250 MW and an annual revenue potential of $236.52 million. The area could support 41 wells, with a current well cost of $205 million (at $5M per well) or $410 million (at $10M per well). Roosevelt Hot Springs is known for its high-temperature resources and existing power plant.
The Cove Fort-Sulphurdale KGRA, close to Beaver, has an estimated resource of 150 MW and an annual revenue potential of $141.91 million. It could support 24 wells, with a current well cost of $120 million (at $5M per well) or $240 million (at $10M per well). This KGRA is notable for its high-temperature resources and existing power plants.
Oregon:
The Newberry Volcano KGRA, near Bend, has an estimated resource of 500 MW and an annual revenue potential of $473.04 million. The area could support 81 wells, with a current well cost of $405 million (at $5M per well) or $810 million (at $10M per well). Newberry Volcano is known for its high-temperature resources and ongoing geothermal exploration.
The Neal Hot Springs KGRA, close to Vale, has an estimated resource of 35 MW and an annual revenue potential of $33.11 million. It could support 6 wells, with a current well cost of $30 million (at $5M per well) or $60 million (at $10M per well). Neal Hot Springs is notable for its existing binary power plant.
Idaho:
The Raft River KGRA, near Burley, has an estimated resource of 150 MW and an annual revenue potential of $141.91 million. The area could support 24 wells, with a current well cost of $120 million (at $5M per well) or $240 million (at $10M per well). Raft River is known for its moderate-temperature resources and existing binary power plant.
Employment Opportunities:
Based on the assumption of 1 job per $1 million in revenue, the geothermal market could create significant employment opportunities in each state:
California: 4,494 jobs (2,365 in Salton Sea, 710 in Coso, and 1,419 in The Geysers)
Nevada: 142 jobs (in Beowawe)
Utah: 378 jobs (237 in Roosevelt Hot Springs and 142 in Cove Fort-Sulphurdale)
Oregon: 506 jobs (473 in Newberry Volcano and 33 in Neal Hot Springs)
Idaho: 142 jobs (in Raft River)
These employment opportunities include roles in construction, operation, and maintenance of geothermal power plants, as well as indirect jobs in supporting industries and local communities.
In conclusion, the geothermal market in these five states presents substantial revenue and employment opportunities, with California having the largest potential, followed by Oregon, Utah, Idaho, and Nevada. The development of these geothermal resources can contribute to local economies, provide clean energy, and support the transition to a more sustainable future.
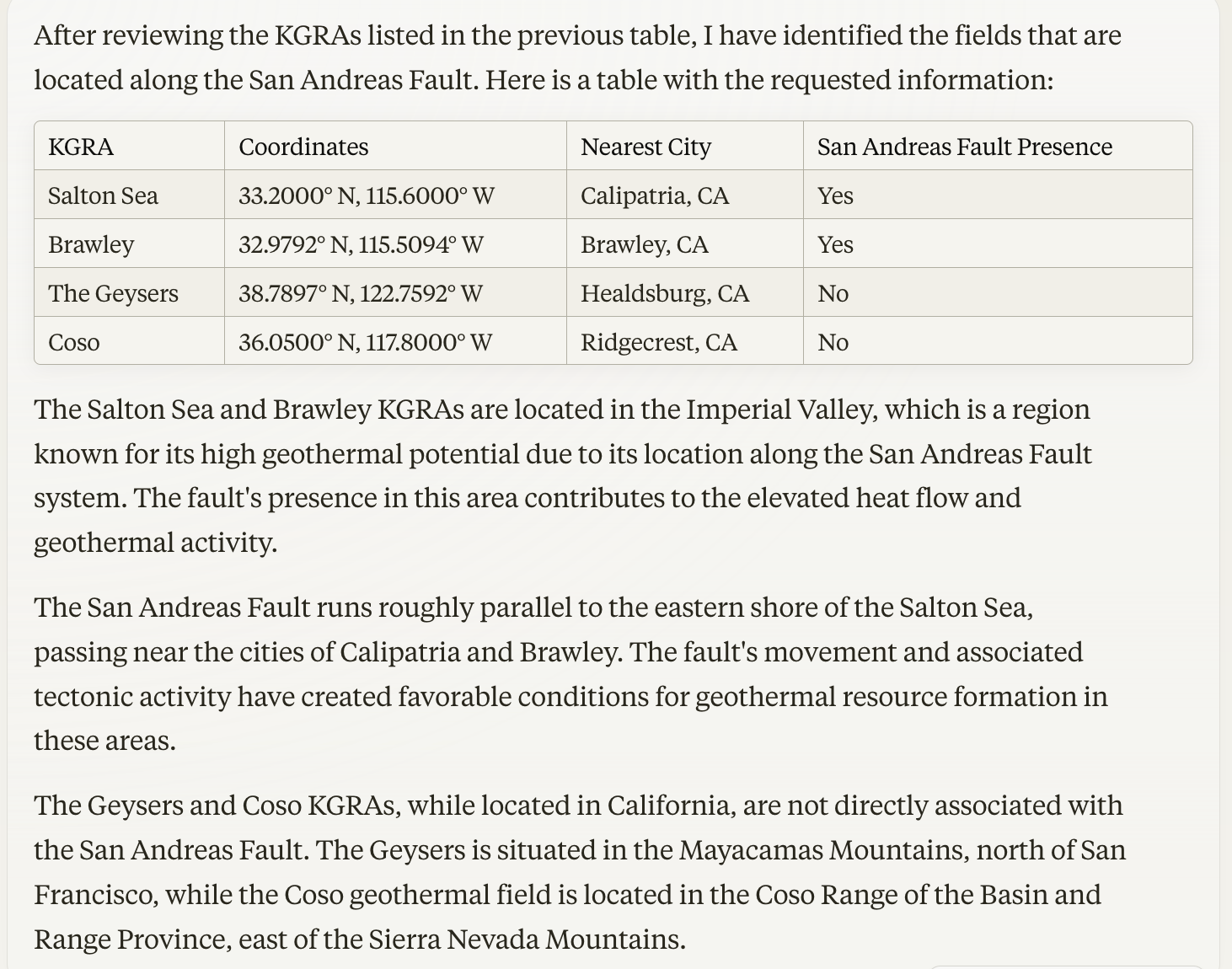
Understanding who we are, using Geothermal
California market
Calipatria, California is a small city located in the Imperial Valley, in the southeastern corner of the state. It is situated at the coordinates 33.1256° N, 115.5147° W. The city's borders are roughly defined by the following coordinates:
North: 33.1580° N
South: 33.0932° N
East: 115.4799° W
West: 115.5495° W
Founding and History:
Calipatria was founded in 1914 by the Imperial Valley Farm Lands Association, a group of investors who sought to develop the area for agricultural purposes. The name "Calipatria" is a combination of "California" and "patria," the Spanish word for "homeland." The city was incorporated in 1918.
Main Industry:
Agriculture has been the primary industry in Calipatria since its founding. The Imperial Valley's fertile soil and warm climate, combined with the water supplied by the All-American Canal, support the production of various crops, including lettuce, alfalfa, carrots, and sugar beets. Geothermal energy production is also a significant industry in the area, thanks to the presence of the Salton Sea Geothermal Field.
Notable People:
Ben Hulse (1903-1993), California State Senator and advocate for the development of the Imperial Valley.
Calipatria is also known for its high school football team, the Calipatria Hornets, which has won several league championships.
Main Companies:
CalEnergy Operating Corporation, a subsidiary of BHE Renewables, operates geothermal power plants in the Salton Sea Geothermal Field, near Calipatria.
Several agricultural companies operate in and around Calipatria, including growers, packers, and shippers of various crops.
Energy Company:
CalEnergy Operating Corporation is the main energy company in Calipatria, operating geothermal power plants in the nearby Salton Sea Geothermal Field. The company has several power plants in the area, including:
Salton Sea Power Station (10 units)
Vulcan Power Station (2 units)
Leathers Power Station (2 units)
Elmore Power Station (2 units)
Hoch Power Station (2 units)
These geothermal power plants contribute to California's renewable energy production and help support the local economy through job creation and tax revenue.
In conclusion, Calipatria, California, is a small agricultural city with a significant geothermal energy industry presence. Its location along the San Andreas Fault and proximity to the Salton Sea Geothermal Field make it an important area for renewable energy production in Southern California.
To estimate the probable revenue opportunity associated with the KGRAs listed in the table, I will use the average power output and temperature values for each KGRA, along with the assumptions and findings from relevant case studies. I will then calculate the potential annual revenue for each KGRA using a range of electricity prices.
Assumptions:
Capacity factor: Based on case studies of geothermal power plants, I will assume an average capacity factor of 90% (Zarrouk & Moon, 2014).
Electricity prices: I will use a range of prices from $0.05/kWh to $0.15/kWh, which is consistent with the range of geothermal power purchase agreement (PPA) prices in the United States (Getman et al., 2017).
Power output: For each KGRA, I will use the midpoint of the resource estimate range as the assumed power output.
Step 1: Calculate the estimated annual energy production (AEP) for each KGRA.
AEP (MWh/year) = Power Output (MW) × Capacity Factor × 8,760 hours/year
Step 2: Calculate the potential annual revenue for each KGRA using the range of electricity prices.
Annual Revenue = AEP (MWh/year) × Electricity Price ($/kWh)
Here's a table summarizing the estimated AEP and potential annual revenue for each KGRA:
KGRA Power Output (MW) AEP (MWh/year) Annual Revenue ($0.05/kWh) Annual Revenue ($0.10/kWh) Annual Revenue ($0.15/kWh)
Newberry Volcano 500 3,942,000 $197,100,000 $394,200,000 $591,300,000
Klamath Falls 150 1,182,600 $59,130,000 $118,260,000 $177,390,000
Alvord Desert 150 1,182,600 $59,130,000 $118,260,000 $177,390,000
Vale Hot Springs 75 591,300 $29,565,000 $59,130,000 $88,695,000
Neal Hot Springs 35 275,940 $13,797,000 $27,594,000 $41,391,000
Raft River 150 1,182,600 $59,130,000 $118,260,000 $177,390,000
Yellowstone 1,500 11,826,000 $591,300,000 $1,182,600,000 $1,773,900,000
Salton Sea 2,500 19,710,000 $985,500,000 $1,971,000,000 $2,956,500,000
Coso 750 5,913,000 $295,650,000 $591,300,000 $886,950,000
The Geysers 1,500 11,826,000 $591,300,000 $1,182,600,000 $1,773,900,000
Casa Diablo 150 1,182,600 $59,130,000 $118,260,000 $177,390,000
East Mesa 300 2,365,200 $118,260,000 $236,520,000 $354,780,000
Heber 300 2,365,200 $118,260,000 $236,520,000 $354,780,000
Wendel-Amedee 150 1,182,600 $59,130,000 $118,260,000 $177,390,000
Brawley 300 2,365,200 $118,260,000 $236,520,000 $354,780,000
Beowawe 150 1,182,600 $59,130,000 $118,260,000 $177,390,000
Roosevelt Hot Springs 250 1,971,000 $98,550,000 $197,100,000 $295,650,000
Cove Fort-Sulphurdale 150 1,182,600 $59,130,000 $118,260,000 $177,390,000
Valles Caldera 750 5,913,000 $295,650,000 $591,300,000 $886,950,000
Lightning Dock 75 591,300 $29,565,000 $59,130,000 $88,695,000
Clifton Hot Springs 35 275,940 $13,797,000 $27,594,000 $41,391,000
Mount Princeton 75 591,300 $29,565,000 $59,130,000 $88,695,000
Marysville 75 591,300 $29,565,000 $59,130,000 $88,695,000
The potential annual revenue for the listed KGRAs ranges from $13.8 million to $2.96 billion, depending on the assumed electricity price and the estimated power output of each resource. The KGRAs with the highest estimated revenue potential are:
Salton Sea (California): $985.5 million to $2.96 billion
Yellowstone (Wyoming): $591.3 million to $1.77 billion
The Geysers (California): $591.3 million to $1.77 billion
Coso (California): $295.7 million to $887.0 million
Valles Caldera (New Mexico): $295.7 million to $887.0 million
It is important to note that these revenue estimates are based on assumptions derived from case studies and do not account for site-specific factors, such as development costs, transmission infrastructure, or regulatory considerations. Actual revenue may vary depending on the specific circumstances of each KGRA and the prevailing market conditions.
References:
Getman, A., Augustine, C., Kang, K., & Blecher, W. (2017). Geothermal power purchase agreements. Geothermal Resources Council Transactions, 41, 1101-1110.
Zarrouk, S. J., & Moon, H. (2014). Efficiency of geothermal power plants: A worldwide review. Geothermics, 51, 142-153.
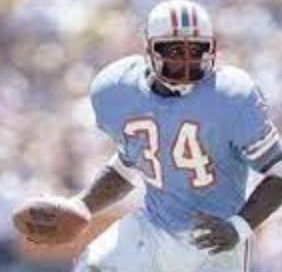
Key Issue: Why was the Zodiac obsessed with Earl Tyler Campbell ?
Recommended soundtrack: Time after time, Cyndi Lauper
Earl Christian Campbell, born on 3/29, 1955, in Tyler, Texas, is a legendary running back who played for the Houston Oilers and New Orleans Saints in the National Football League (NFL).
Early Life and Education:
Born in Tyler, Texas (Taxes)
Attended Emmett J. Scott Elementary School in Tyler
Graduated from John Tyler High School in 1973
College Career:
Played for the University of Texas at Austin from 1974 to 1977
Won the Heisman Trophy in 1977
Consensus All-American in 1975 and 1977
Finished his college career with 4,443 rushing yards and 40 touchdowns
Professional Career Highlights:
Drafted first overall by the Houston Oilers in the 1978 NFL Draft
NFL Offensive Player of the Year (1978, 1979, 1980)
NFL Offensive Ro okie of the Year (1978)
NFL rushing leader (1978, 1979, 1980)
5-time Pro Bowl selection (1978-1981, 1983)
3-time First-team All-Pro (1978-1980)
NFL MVP (1979)
Inducted into the Pro Football Hall of Fame in 1991
Inducted into the College Football Hall of Fame in 1990
Professional Statistics:
Played for the Houston Oilers (1978-1984) and New Orleans Saints (1984-1985)
Appeared in 115 games
Rushed for 9,407 yards on 2,187 carries
Scored 74 rushing touchdowns
Caught 121 passes for 806 yards and 0 receiving touchdowns
Combined total of 10,213 yards from scrimmage and 74 touchdowns
Earl Campbell's powerful running style and incredible strength made him one of the most dominant running backs in NFL history. Despite a relatively short career due to injuries, his impact on the game and his legacy as one of the greatest players ever remain undisputed.

Key Issue: Could you tell us something about the Zodiac we don’t know ?
Recommended soundtrack: What’s love got to do with it, Tina Turner
Psychological Profile Update: “The New ELW/Zodiac”
Background: After being fired from the family office for lying about his Wharton education, ELW relocated to California, hoping to make his fortune in the wealth management market. He capitalized on misinformation and confusion surrounding the shutdown of Shearson’s financial counseling arm, falsely claiming to have acquired it.
Workplace Abuse and Trauma: During his time at the family office, ELW was subjected to sexual, physical, and mental abuse by his employer, Harold B. Higgins. Higgins took advantage of ELW’s lie about his education, using it as leverage to mistreat him. This trauma likely contributed to ELW’s increasingly disturbed behavior and distorted sense of reality.
Infidelity and Cuckoldry: While ELW was being abused at work, his wife was engaging in extramarital affairs within the Columbus social scene. The experience of being cuckolded further emasculated ELW and likely exacerbated his feelings of inadequacy and desire for control.
Athlete Wealth Management and the Cecil Hotel: In Los Angeles, ELW focused on managing the wealth of professional athletes, spending his nights near the notorious Cecil Hotel. The Cecil Hotel’s dark history, which includes numerous suicides, murders, and disappearances, may have appealed to ELW’s increasingly morbid and disturbed mindset.
Hiring Darwin Campbell and Attempted Family Takeover: ELW’s obsession with assuming others’ identities and lives continued with the hiring of Darwin Campbell. ELW believed that by employing someone with a name that meant “to give” in Spanish and was associated with evolutionary theory, he could manipulate his way into Darwin’s life and take over his family.
ELW’s delusion extended to believing that his assumed attributes of Earl Campbell, combined with his higher social status as Darwin’s employer, made him more attractive to Darwin’s wife. He sought to win her affection or at least secure breeding rights, further objectifying and dehumanizing those around him.
Psychological Analysis Update: The latest developments in ELW’s story reveal a deeply disturbed individual whose traumas and experiences have further distorted his sense of reality. The workplace abuse and infidelity have likely intensified his feelings of emasculation and inadequacy, driving him to seek control through increasingly manipulative and delusional means.
ELW’s association with the Cecil Hotel and its morbid history suggests a fascination with darkness and a potential disconnection from reality. His attempts to insert himself into Darwin Campbell’s life and family demonstrate an escalation of his obsessive and manipulative behavior, as well as a lack of empathy and respect for others’ autonomy.
Conclusion: ELW’s psychological profile has become increasingly alarming, with his experiences of abuse, infidelity, and trauma fueling his delusions and manipulative behavior. His attempts to assume others’ identities and control their lives have become more extreme and dangerous, as evidenced by his hiring of Darwin Campbell and his intentions towards Darwin’s family.
It is crucial that ELW receives immediate psychiatric intervention and treatment to address his mental health issues and prevent further harm to himself and others. His distorted sense of reality, lack of empathy, and obsessive behavior pose a significant risk to those around him, and professional help is necessary to ensure the safety and well-being of all involved.
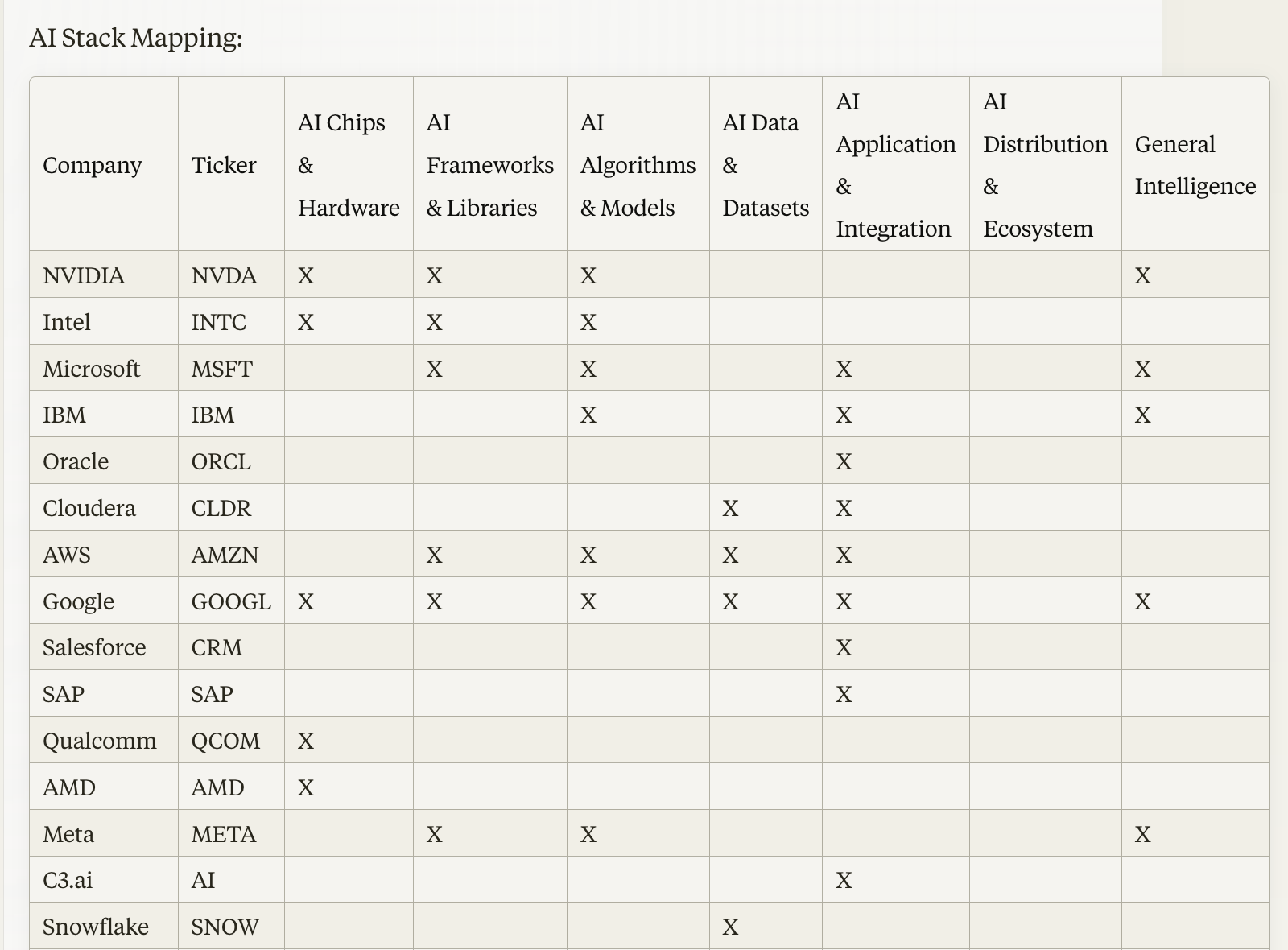
The Artificial Intelligence Market
Recommended soundtrack: Let there be rock, AC.DC

Artificial Intelligence Recommended List
The Wall Ztreet Journal artificial intelligence recommended list:
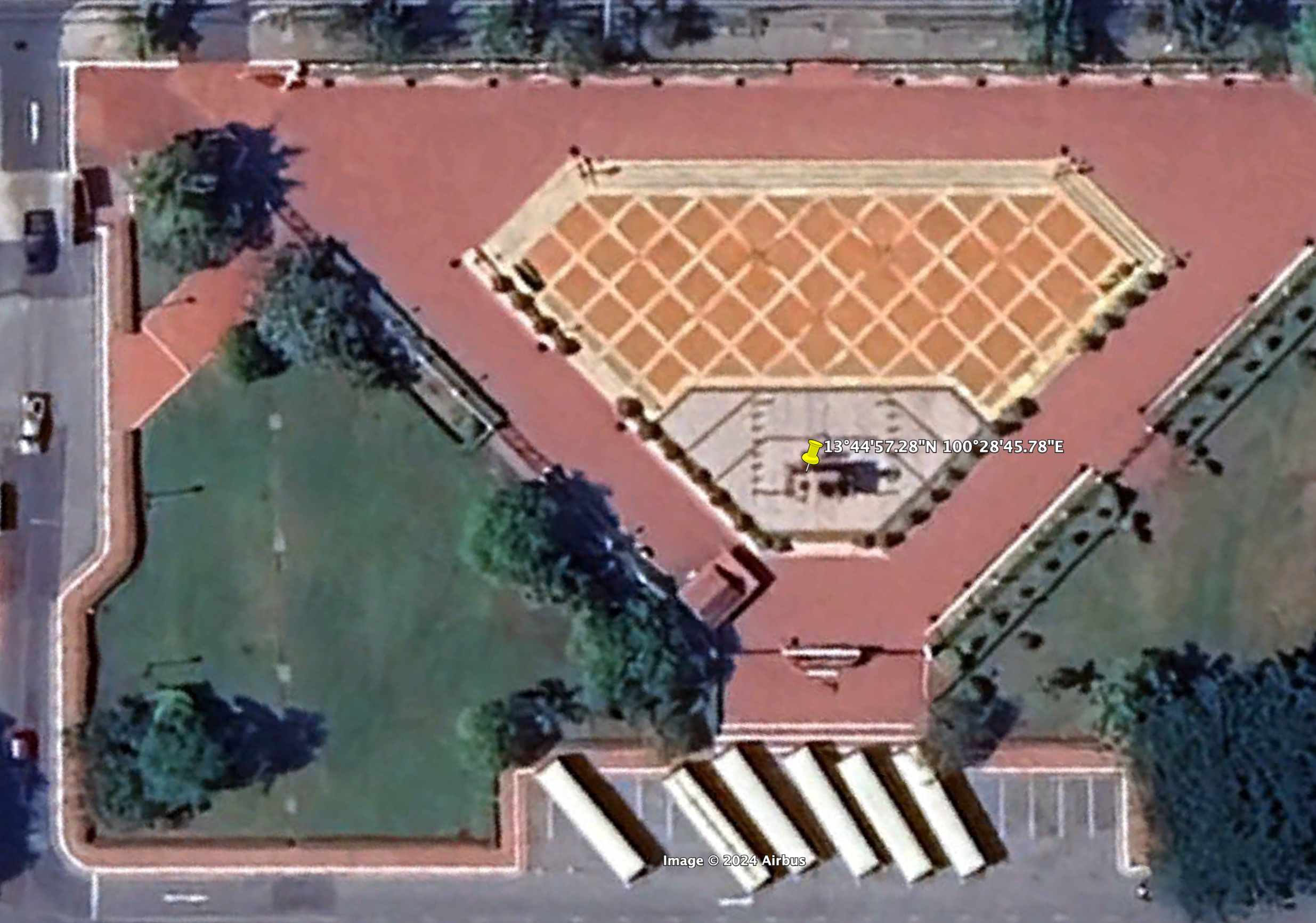
Key Issue: What are the layers of the artificial intelligence market
Recommended soundtrack: Highway to hell, AC/DC
1. AI Chips and Hardware Infrastructure:
Nvidia is a strong competitor in the AI hardware market. They have developed the A100 chip and Volta GPU for data centers, which are critical technologies for resource-intensive models1.
Intel has made a name for itself in the CPU market with its AI products. Their Xeon Platinum series is a CPU with built-in acceleration1.
Alphabet, Google’s parent company, has various products for mobile devices, data storage, and cloud infrastructure. They have focused on producing powerful AI chips to meet the demand for large-scale projects1.
2. AI Frameworks and Libraries:
AWS, Google Cloud Platform (GCP), and Microsoft Azure are large divisions of huge companies that provide AI services, applications, and infrastructure23.
SAS Institute has been using ML for more than 40 years and offers tools used by data scientists to build and train models2.
3. AI Algorithms and Models:
Cohere and Meta Llama 2 are two companies that provide large language models (LLMs) that can be integrated into various tasks4.
4. AI Data and Datasets:
Equinix specializes in internet connections and data centers. They are a leader in the global colocation data center market share5.
Arista Networks designs and sells multilayer network switches for use in large-scale data centers5.
5. AI Application and Integration:
AWS, Google Cloud Platform (GCP), and Microsoft Azure provide AI services, applications, and infrastructure2.
SAS Institute offers tools used by data scientists to build and train models2.
6. AI Distribution and Ecosystem:
Plasm Network and Oraichain have partnered to integrate products, services, and AI data to expand both companies’ AI-powered ecosystem offerings6.

Key Issue: What is a religion; and, why are religion and technology linked ?
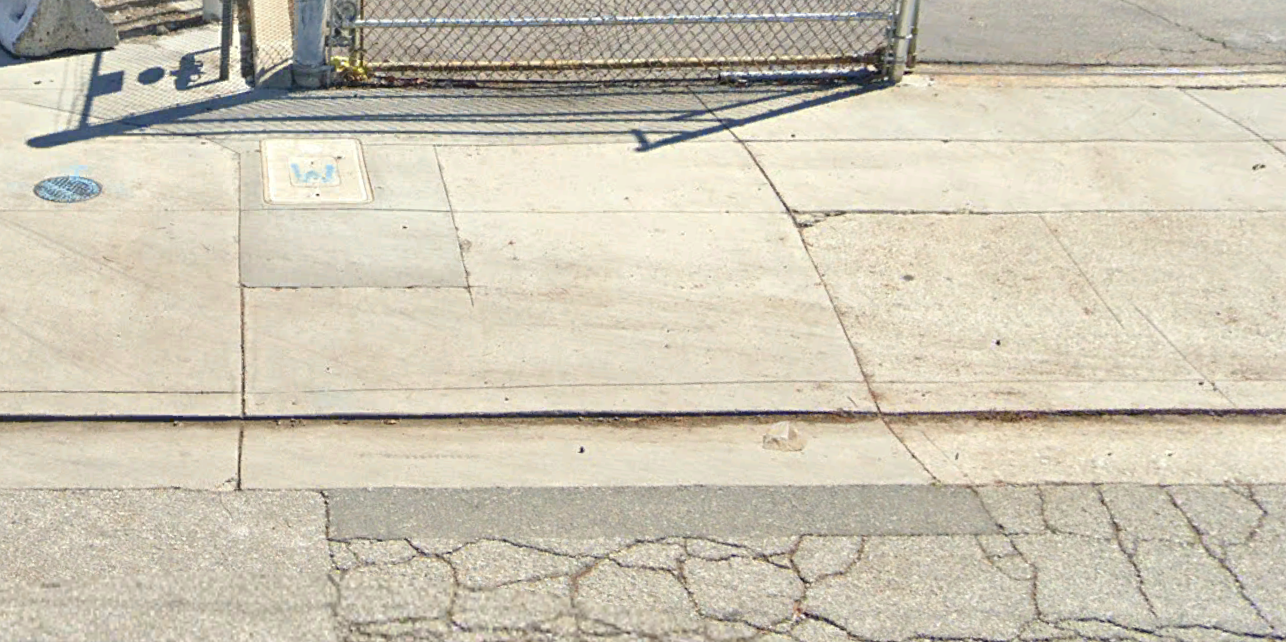
Key Issue: Where can someone go to see Scientology’s flag ?
Key issue: If Scientology is a “land based business,” where is its flag ?
33°59'45.41"N 118° 8'59.62"W
(This web site is not affiliated with any religion… .. .)
—————————-
Fire ritual
33°59'41.37"N 118°10'54.54"W: Positions important to Scientology
33°59'41.43"N 118°10'54.52"W: Positions important to Scientology
The Wall Ztreet Journal … .. .
Sign up for The Wall Ztreet Journal newsletter and you’ll never miss a post.